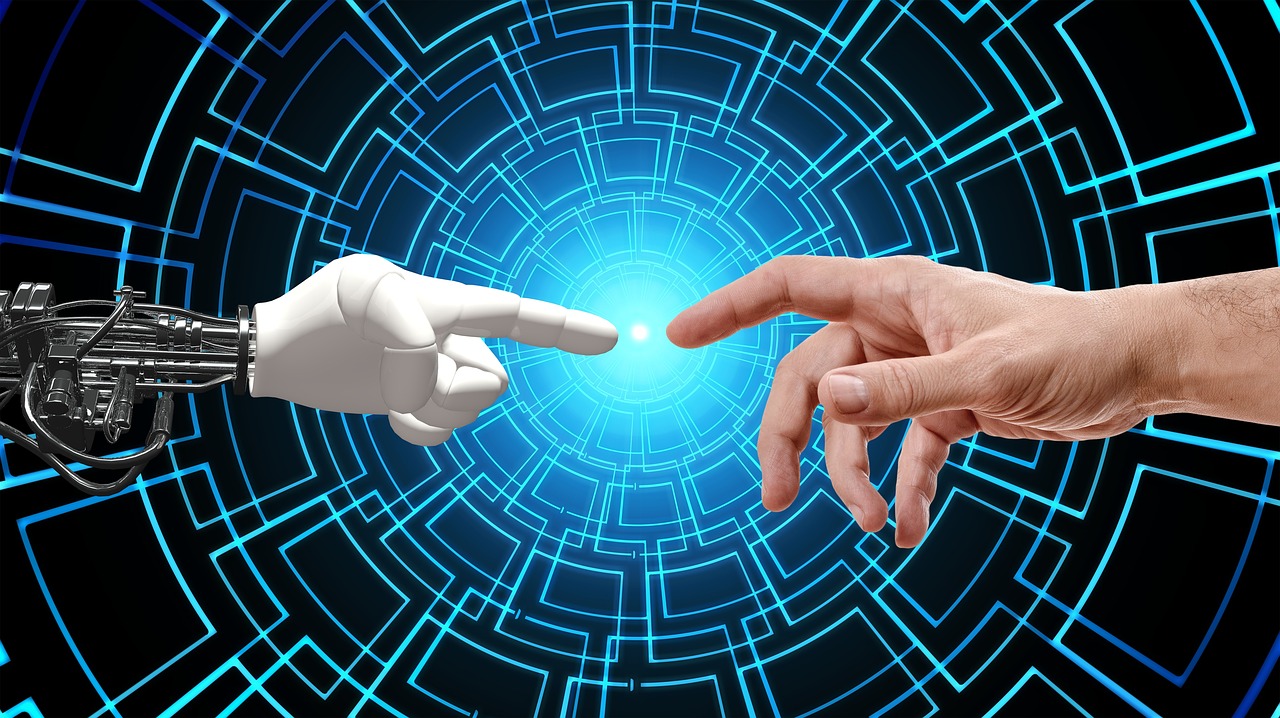
Machine Learning – The Best Defense Against Chargebacks
Aug 7, 2019
Machine learning has become an excellent line of defense for high-risk merchants tackling fraud and chargebacks. It was once reserved for the worlds of academia and supercomputing. Presently, its broad range of applications are gradually realized considering the operational costs of high-performance computing has decreased. This is particularly true in the payments world.
Today, payment processors already take advantage of its capabilities every day. This includes activities like monitoring credit card transactions, using machine learning algorithms that assess up to 50 data points to authorize payments in real-time.
As a high-risk merchant, machine learning and artificial intelligence (AI) provides an opportunity to fight back against your ever-increasing credit card fraud losses. Damages are set to exceed $30 billion globally by 2020. Using machine learning to reduce fraud and chargebacks can help build up processing history. It can also open the door to new payment options and improved operating capacities.
What is machine learning?
Machine learning is an evolving subcategory within the broader category of AI. Traditional algorithms operate on rules-based systems developed by their human programmers. Machine-learning algorithms are designed to learn from their experiences. Therefore, its performance improves over time without external human input.
The banking and payment industry has used this technology as a weapon against online criminals attempting to commit fraud through e-commerce merchants. This is particularly the case for card-not-present fraud, which has soared in recent years after the increased security of EMV technology.
Machine learning in e-commerce payment processing terms is the ability to establish behaviors and patterns that indicate a transaction is likely to be fraudulent. By analyzing dozens of data points on an on-going basis, algorithms can gradually increase their potency when it comes to spotting irregularities. They help to develop a so-called “eDNA” of each buyer, which spots anomalies quickly, and marks a transaction as fraudulent before payment is taken. This development has also led to advancements of authentication technology, by prompting customers for more data before completing their transactions.
PSD2 and 3DS2 are forms of fraud prevention
The two most commonly used authentication tools are PSD2 and 3DS2, since usernames and passwords are becoming increasingly insecure.
PSD2 requires customers to provide information from two of three categories which are knowledge, possession, and inherence. In other words, shoppers need to provide the following:
- something only they have (credit card or cell phone);
- something only the customer knows (answer to a secret security question); or
- something they are (fingerprint or facial scan).
The second version of 3D-secure is 3DS2. The original 3D-secure had growing pains. Website redirection and a requirement for original card issuer details to be entered were formerly considered a conversion killer. The new 3DS2 streamlines the process by operating a SMS two-factor authentication process. At the same time, it sends the remaining data points surrounding the transaction to the bank or issuing provider. They then use their own machine learning algorithms to detect whether it’s fraudulent or legitimate, shifting the liability away from you, the merchant.
What kind of fraud can machine learning help prevent?
Machine learning can prevent fraud in a multitude of ways, giving extra protection to merchants across the globe. Below are the five most common frauds and how machine learning is instrumental is detecting and preventing them:
1. Duplicate Transactions
Duplicate transactions are a common issue whereby a customer enters payment information and authorization takes quite long, so the user refreshes the order. The result is two orders and a chargeback for the merchant down the road for one of the payments. Through AI, human errors can be instantly discovered and reliably distinguished (e.g. double-clicking a button). In turn, it can block duplicate transactions and decrease crucial chargeback ratios.
2. Identity/Credit Card Theft
Identity theft occurs when a scammer sets up a user account with someone else’s information (usually after stealing their credit card). The fraudster extracts money or goods from an e-commerce merchant using that stolen information. Smart algorithms use behavior analytics to search and uncover those inconsistencies in personal data sets and block the transaction.
3. Friendly Fraud
Friendly fraud is the result of a customer issuing a chargeback rather than asking for a refund. This is usually because they didn’t understand what they were signing up for or don’t recognize your company name on their credit card statement. Machine learning can benefit the dispute process by proving a purchase was intentional through detecting data-like fingerprints. It also assists in automating responses to friendly fraud chargebacks. Since the reasons behind these frauds are often similar, AI improves turnaround times on disputes as it learns the most common responses and how to deal with them appropriately.
4. Account Hacking
Account hacking is similar to identity theft and occurs when a customer’s user information is compromised. Therefore, all the information entered into an e-commerce merchant’s site is correct when trying to make fraudulent purchase. Once again AI algorithms analyze dozens of data points. Devices, cookies, IP addresses, time of day, and network to stop transactions that don’t match previous buying behavior are just some examples.
5. Chargeback Fraud
Chargeback fraud is almost identical to friendly fraud. It occurs when a user maliciously issues a chargeback knowing they already consumed the product. With the onset of 3DS2 and other data protocols, machine learning algorithms can scan data points for previous malicious activity. This direct action can frequently be associated with a customer’s old credit card number. The direct result is a blocked order from the potential client. Moreover, if the completed transaction does pass all checks, machine learning tools can prove the service or product was used. Therefore, this evidence can support you in the chargeback dispute process. Also things like verifying customer logs and shipping details help combat this type of fraud. Due to AI advancements, the dispute process can also be automated (to an extent).
Combat existing high levels of fraud
When it comes to implementing machine learning, there are multiple ways to begin reducing risk and chargeback levels that are considered acceptable to payment processors. Firstly, you can request access to your bank’s or gateway’s built-in anti-fraud tools. They should be willing to cooperate since any reduction in your fraud rates are mutually beneficial. Additionally, authentication tools are excellent for stop suspicious transactions in their tracks. PSD2 and 3DS2 checks are set to significantly decrease online fraud rates when they come into effect later this year.
Furthermore, you can customize in-house shopping carts and CRMs to start collecting as much data (with consent) as possible concerning your customers. The more data you manage to collect, the better machine learning algorithms will be at detecting anomalies or unusual buyer behavior.
Lastly, several third-party machine learning analytics tools, applications and plugins can be installed on your website to recognize customer behavior patterns. This will help increase sales. Additionally, expect fraud detection rates to rise concurrently as you set rules for algorithms to decline transactions that have followed certain previously suspicious patterns. Start off with a few rules and build more in to avoid any false negatives which can result in sales losses.
Example of how machine learning is effective for high-risk merchants
Machine learning in the e-commerce industry has many applications that can help high-risk merchants turn their processing history around. Even by setting simple rules, AI helps algorithms to learn and improve their implementation, gradually increasing fraud detection rates and lowering chargebacks.
For example, you could set up a rule to block any transactions attempted with multiple credit cards bearing the same customer name and IP address. With data collected from your shopping cart, CRM or other sources, the algorithm may work out extra details. This can include finding the type of fraudulent activity most common for female customers aged 18-36 with a Florida address.
By learning these customer traits, the algorithm can automatically flag suspicious transactions that go far beyond your initial rules of engagement. Thus preventing far more fraudulent transactions than you ever would have, had you programmed the algorithm yourself.
With the help of algorithms, e-commerce merchants can slash their rates of fraud and chargebacks helping them to apply for increases in monthly payment processing capabilities.
Incorporate machine learning into your business today
As you can see there are big benefits from harnessing the power of AI and machine learning. From reduced fraud, lower chargeback rates, and improved payment processing history, using machine-learning algorithms is one of the fastest ways to impress payment processors as an e-commerce merchant.
Although the technology sounds complicated, user interfaces are often simple to operate. You can lower costs by sharing information with mutually beneficial parties, such as your bank. So don’t delay, start experimenting and implementing machine learning today.
Does your credit card processing strategy need a complete overhaul? Is your e-commerce business drowning in fraud and chargebacks? Then make sure to contact our team at DirectPayNet to regain control over your e-commerce business.